Reliability connect | BY Ken Madsen
Implementing IIoT-Based Asset Reliability Program at a Gold Mine
The wireless Predictive Maintenance program paid for itself within six months at a leading gold mining company. They demonstrated increases in asset reliability, personnel efficiency, and production as part of their digital transformation initiative.
STARTING WITH A DIGITAL FIRST MINDSET
One of the world’s largest gold mining companies in the world is undergoing a digital transformation to integrate data and technology throughout their operations. Part of the objective is to get the right information to the right people at the right time and to connect disparate operations. The digital transformation team identified an opportunity to get better machine health data, more often, in real time, by working with Petasense, an Industrial IoT startup.
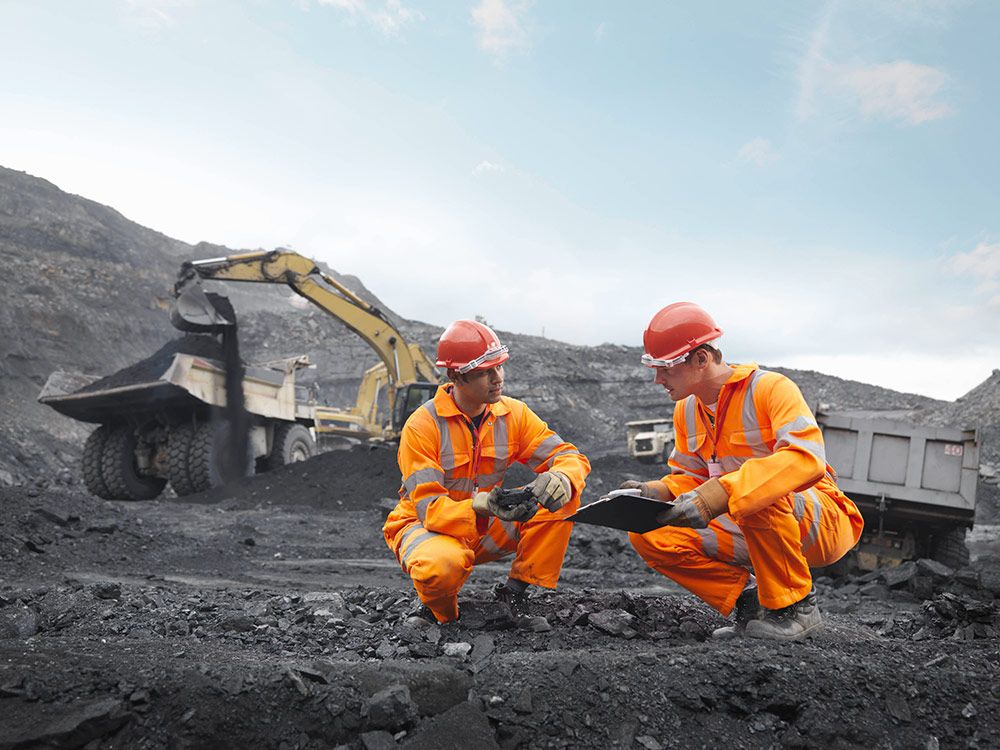
With a digital first mindset, the mining customer implemented Petasense’s Asset Reliability & Optimization (ARO) system and wireless sensors to monitor several critical pumps, fans, SAG and Ball mills in one facility. They had an existing Predictive Maintenance (PdM) program in place for over 20 years that relied on walkaround vibration data collection, and they identified opportunities to reduce failures further by increasing the frequency of data collection.
The challenge with walkaround programs is problems that develop during the intervals can be missed. Even with an experienced team, several failures had developed quickly in between monthly and quarterly routes, causing unplanned downtime.
EFFICIENCY, INTEGRATION, AND RUGGEDNESS
The Petasense Vibration Motes (permanently installed wireless vibration sensors) collect data automatically, and the data is analyzed with advanced machine learning algorithms to predict equipment problems. Each machine is assigned an asset health score and provides real-time alerts when the score falls below an acceptable number. This allows the analyst to focus only on problem equipment, with access to all the sophisticated vibration analysis features that they are used to using.
Using Petasense Motes, the customer was able to instrument remote assets that are difficult to access for regular vibration readings. Another important factor for selection was ruggedness of the Motes (IP67 rated) in the dusty, harsh mining and mineral processing environment.
The customer has been using OSIsoft Pi data historian for many years, and they didn’t want another standalone system. They appreciated Petasense’s open architecture that easily integrates with Pi. Vibration readings are brought into Pi, and the team uses this to analyze process and reliability data simultaneously to make better decisions and optimize equipment performance.
DEMONSTRATING ROI FROM REAL-TIME MONITORING
Petasense’s implementation was completed within a few weeks. This included installation of the Motes, configuration of the ARO Cloud software and Pi integration. Soon after going live, the PdM team was alerted by high vibrations on the drive end of a Mercury Scrubber Fan. The high vibration generated real-time alerts which allowed the maintenance team to shut it down proactively and fix the problem.
After troubleshooting for fan imbalance and visually inspecting, the PdM team determined that there were loose frame bolts holding the motor and fan frame. The looseness had caused structural damage and broken the weld in frame.
The Mercury Scrubber Fan helps with recovery percentage, and a failure would mean shutting down the kiln. While it doesn’t shut down the plant, it can help gold recovery by 1-2%, and over the long term, this can cause millions of dollars in lost revenue. Before working with Petasense, the customer had experienced a failure on the same fan and it was offline for six weeks, waiting for the fan assembly to arrive and causing significant production losses.
Another win for the PdM team was high-flow centrifugal pumps in the heap leach field. The heap leaching operations are used to recover lower-grade oxide ore. They consist of applying a leaching solution to piles of crushed ore to extract the gold. The customer had increased the amount of gold extracted, to the point that both the primary and standby pumps were needed to get the flow rates and the tank was undersized for current demand.
With the Petasense Motes, they were able to diagnose cavitation in the pumps. A failure on these pumps lowers the gold recovery amount. Knowing this, the PdM team were able to identify the need for a design change to increase reliability.
EXPANDING THE PREDICTIVE MAINTENANCE PROGRAM
The early success of detecting failures helped justify expansion of the program to other rotating assets and sites, as well as multi-parametric monitoring. Machine monitoring is limited when just a singular parameter, such as vibration, is used. It’s most effective when combined with related parameters such as load or running speed. By installing the Petasense Transmitter, the customer was able to wirelessly collect data from hundreds of different types of sensors, including temperature, pressure, ultrasound, current, vibration, and more.
In addition to expanding the program to more types of data, the PdM team has identified other assets that are not monitored today. They are planning to install Motes on critical equipment like conveyors, which the PdM team cannot access with normal route-based data collection. Some of these are critical, non-redundant assets that will take down the production line, making them prime candidates for Petasense wireless sensors.
Using Petasense, the PdM team was able to demonstrate tangible results that aligned perfectly with their digital transformation. One of the success factors was the true partnership between the corporate digital transformation team and plant personnel responsible for implementing and using the information. They were able to successfully navigate the culture change by picking technologies that demonstrated fast Return on Investment to both the shop floor and the digital team.